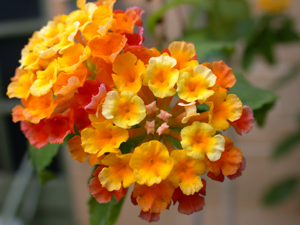
|
BIOL 3110
Biostatistics
Phil Ganter
302 Harned Hall
963-5782 |
Lantana flowers-
notice the older, outer
florets are darker in color |
Sampling Distributions
Chapter 5 (skip
sections 5.1 and 5.2 in 3rd ed.)
Email
me
Back to:
Unit
Organization:
Problems:
Problems for homework
- 5.11, 5.12,
5.18, 5.20, 5.24, 5.33, 5.34, 5.35, 5.43, 5.45
- 4th edition
- 5.2.1, 5.2.2, 5.2.8, 5.2.10, 5.2.15, 5.4.2, 5.4.4, 5.4.5, 4.S.5
Suggested Problems
- 5.38, 5.45,
5.50. 5.56
- 4th edition
-
5.4.9, 5.S.5, 5.S.8, 5.S.12
Samples and Sample
Variability
Sampling
Variation
Variation among samples of observations drawn from
a single population
- samples differ from one another and from the
true population value due to random chance (if they differ for any other reason
than chance, then the sample is a BIASED sample).
- the
statistics we have been concerned with are the mean of the sample (
, x-bar,
for continuous data) or the frequency of success (
, p-hat, for dichotomous
categorical data) in the sample
Sampling Distribution
Probability of the possible outcomes for
a statistic based on a sample taken from a from a population
- since all possible outcomes are included, the
sum of their probabilities (= total area under curve) must be equal to 1.00
Error, Variation, and Mistakes
- We
expect that, when we choose members of the population to be in a sample,
that they will differ from one another with respect to whatever we are
measuring.
- Understanding
this discrepancy requires that we define two ideas, variation and
error. In addition, error is often used to mean a mistake in common
speech, so we need to separate statistical error from this sort of
error.
- MISTAKES are incorrect choices made by individuals and
we will keep this idea separate from that of statistical error
by using mistake for poor choices and error only in the statistical
sense.
- VARIATION is the difference among members of the population
under study.
- Standard
Deviation measures the degree of difference among
members of the population.
- Notice
that, after reading about error below, each data point
can be affected by measurement error and so standard deviations
reflect both the true differences among experimental units
and any measurement error associated with collecting the
data.
- ERROR arises when there is a difference between an estimated
value and some actual (=true) value.
- This
error is not error in the sense of a mistake but is an unavoidable
consequence
of our methods (part of the structure of our world).
- MEASUREMENT
ERROR is
caused by the inaccuracy of our measurement method. Anyone who
has used a balance knows about this sort of error.
- In the book, this is called NONSAMPLING
ERROR, but it is the same. They use examples from survey data and, in
this case, the survey is the measurement method.
- The book refers to survey problems like
non-response bias, but we will not deal with these problems here. Using
survey data has long been studied by sociologists and is too deep for
us in this course.
- Notice that each data point will be affected
by this sort of error.
- SAMPLING ERROR is
caused by the inaccuracy introduced when using a sample instead of the
entire population.
- When we draw a sample from the population
in a random fashion and calculate a mean from this, we acknowledge that
the sample mean is the best estimate of the population mean, but we also
recognize that it may differ from the actual mean.
- The difference between the sample mean
and the true mean is measured by the standard error and is a form of
statistical error because it is the result of the sampling procedure.
- When error is not the result of a random process,
whether it is measurement or statistical error, it becomes BIAS.
- BIAS is systematic error, error that is not
random.
- If your scale is not zeroed, then all of
the weights you take may be too large. so your estimate of weights is
biased towards over-estimating the weights
- If your sampling procedure is not random,
you may pick individuals who all share some quality, even though not
all members of the population have that quality. Since the sample is
not a true reflection of the differences found in the population, this
is a bias.
- If
a sample is biased and the bias can't be corrected (sometimes measurement
error can be corrected), the statistical tests covered in this course
and in your book are not
applicable.
Meta experiments
We will use the metaexperiment to explore the distribution
of sample statistics
- You
do the same experiment over and over again
- each
time you draw a sample, you calculate the statistic of interest based
only on that sample
- the
meta- experiment's data are the statistics calculated from each of
the individual experiments
- Metaexperiments
can be done with either continuous or discrete
data
- See the end of the lecture for
a consideration of the outcome a metaexperiment with dichotomous outcomes,
the only discrete
variation we will consider
We will use the sampling distribution of metaexperiment
results to estimate the probability that a
sample statistic differs from the true
value (the parameter) by a specified amount.
- how
probable is it that a sample means,
, is
a given distance from
the true mean mean,
,
- how
probable is it that a sample frequency of success,
, is
a given distance
from the true frequency of success,
,
These are instances of drawing
a STATISTICAL INFERENCE.
- a statistical inference is a conclusion
about a population inferred from a sample drawn from that population
- Notice that
statistical inferences never give you a yes-or-no answer, only the
probability
of a
particular
outcome
Metaexperiments
and Sample Size
As the sample size n (also n in the
binomial) increases, the sampling distribution of the x-bar or p-hat becomes
narrower and narrower as there is less variation among the sample statistics
- This can be stated in a common sense manner
as: larger samples are more likely to be an accurate estimate of the
population than are small samples
- The
sample size referred to here is the size of each sample drawn from the
population, not the metapopulation sample size, i.e. the number of time
you draw a sample from the population
- Thus,
in a metaexperiment, all samples drawn from the population must be the
same
size
One implication of this is that, as the
sample size increases, the chance that x-bar or p-hat will be close to the true
mean (mu,
) or chance of success (pi,
) gets greater and
greater
- If you are trying to estimate
the
or
, then a larger sample size will most
likely give you a better estimate than will a small sample size.
- Of
course, there are other considerations (cost, effort, opportunity)
that may affect the actual sample size used in an experimental or observational
study but, in terms of the power of the statistical inference made
with
the data, larger samples are better
Continuous Data
Continuous
data have real-number values associated with them so a sample of these observations
will have a frequency
distribution, mean, median, standard deviation, range, etc.
- The
mean and standard deviation are
the descriptive parameters (introduced in the second chapter) we will
consider
- In
the Continuous Data case, we ask how close to the population parameter
should should our sample statistic be?
- When
a sample mean is calculated, it will usually not equal the true population
mean (although there is a chance that it might be the same) but will
be some distance (let's call it "d" here) from the true mean
- The
most often asked question is how probable is it that the sample mean,
, differs
from the true mean,
, by
a distance equal to or greater than "d"?
- We will concentrate on the mean for most
of the rest of the semester, but you should realize that one can ask this
question of any of the parameters.
- The
answer lies in the Sampling Distribution for that parameter
- Our next step is to find out what that distribution
is.
The Continuous Data MetaExperiment
We have a population
- the mean of the population is =

- the standard deviation of the population is
=

We take repeated samples of size n from the population
and calculate the mean (
, x-bar). This gives us a bunch of x-bars (imagine
thousands of samples are drawn so we have lots of data).
What is the expected mean
of these sample
s?
- The mean of the sample means (
)
should =
. This can be derived most simply through some logical
argument. If random chance is the only reason a sample mean differs
from
, then all
of the
s should be
clustered around
and when you take their average, the random errors
should about cancel out, making the expected average of the sample means
the true mean.
- This
implies that, random chance will be as likely to increase a sample mean
(compared to the true mean) as to decrease it
- This
also implies that (about) half of the sample means will be larger than
the true mean and half will be smaller
- The
"about" in the above statement arises from two sources: random chance is
not precise but probabilistic and some of the sample means might equal
the true mean
What is the expected standard deviation
of these sample means?
- The standard deviation of sample means (
.)
must be related to the variation found in the original population, which
is characterized by the population standard deviation,
, but it is not
simply equal to
.
- Why
is this?
- Well,
in the original population, the values of x had some range from the smallest
to the largest and we use the standard deviation to characterize this variation.
- The
values of the means of samples will also have a range
but is that range likely to be as large as the range of individual values
in the population?
- The
difference is that the variation
among the
sample means should be smaller than the variation among the x values.
- Each
sample mean,
, is calculated
from a sample drawn from the population and will have the effect
of some small
and some large values.
- For
this reason, a sample mean,
, from a randomly
drawn sample should usually
be closer to
, the population mean, than an individual observation
drawn at random from the population.
- So, the sample means
s cluster closer together, near
, than do the members of the population, so
their standard deviation,
,
should be smaller than the population standard deviation, 
- But how much smaller?
- Sample size is
important once again.
- Larger samples should estimate
more closely, that is, the sample means,
, from larger samples should be closer to
than sample
means calculated
from a smaller sample.
- If
large-sample
s are nearer
one another (and
) then their
standard deviation should be smaller.
- So
we need a way to reduce the population standard deviation,
, to
get the standard deviation of the sample means,
, and
that method has to make a larger reduction for big n's (sample sizes)
than for smaller
n's.
- Our
solution:
- Standard
deviation of the sample means =
=
/
What is the shape of the distribution of sample
means?
The
shape of the distribution depends on
- the
shape of the distribution of observations that make up the population
from which samples are drawn
- the
sample size
These
effects can best be summarized as below:
- When
the population is normally distributed, the distribution of
sample means for samples drawn from that population is also normal, no
matter the size of the sample.
- When
the population is not normally distributed, then the shape of the distribution
of sample means depends on sample size
- If the sample size is small (n about 40
or less), then the distribution of sample means for
samples drawn
from the
population will not be normal (it will be similar
to the population distribution)
- If the sample size is large (greater than
40 or so), the shape of the sampling distribution of sample means will
be
normal, no
matter
whether
the population is normally
distributed or not - this is due to the Central Limit Theorem, covered
next
- Thus, problems with normality arise only when the sample
size is small and the population the sample is taken from is not normally distributed
Central Limit Theorem
The distribution of the population has in influence
on the distribution of
s only when the sample size is small.
- As the sample size increases, the distribution
of
s becomes closer and closer to a normal distribution,
no matter what the population's distribution is.
- This is a very good thing for two reasons.
- First, it lets us use the same methods for drawing
inferences about sample means no matter how skewed or kurtotic the original
population distributions are. This makes things simpler than a different methodology
for each distribution
- Second, we know lots of properties of the normal
distribution and can make powerful inferences based on these properties
- However, notice that this is only true for large
sample sizes
- We will see that this means that we need to
approximate the normal distribution, and that the approximation should get
closer to the normal as sample size goes up.
- We
will do this in the next chapter.
So far we have three groups of numbers to keep track
of. Here is a summary of the three (the book does this in Table 5.7)
- The population of x's has a
mean of
and a standard deviation of 
- A sample of size n drawn from
the population has a mean of
and
a standard deviation of s
- Sample means (
), each calculated
from each sample drawn from the population have
a mean of
,
which is expected to be the same as the population mean,
, and a standard
deviation of
which
can be calculated directly as
/
Normal Approximation of the Binomial Distribution
This
section is another outcome from the central limit theorem.
Once again, the binomial has three parameters,
Pr{a success for any particular trial}
=
, j = the number of success, and n = sample size or number
of trials
If
n is large, then the binomial
distribution of outcome probabilities can be approximated by a normal distribution
with
(notice that I use p in this section and the book uses
p, but these are the same value - I want to be more consistent)
- mean = n

- standard deviation = sqrt[n
(1-
)]
If
n is large then the distribution of the probabilities of success (
) in the samples can be approximated with a normal
distribution with:
- mean(p-hat) =

- standard deviation of p' = sqrt[
(1-
)/n]
Note the difference between the
first and second
set of means and standard deviations just presented!
- The first refer to the probability of getting j
successes out of n trials (so the x-axis of the distribution
will go from 0 to j in whole number jumps - remember it's categorical data)
- The second refer to the probability that the proportion
of success in the n trials will be j/n (so the x-axis of the distribution
will be a fraction from 0 to 1)
Why is this useful? Its a time saver when
n gets large
- if n = 200, then what do you
need to calculate the chance of getting more than 50
successes?
- You have to calculate all
of the binomial probabilities for 51 through 200
successes and sum these up.
- You have to calculate all
of the binomial probabilities for 0 through 50 successes,
sum these up, and subtract the sum from 1
- That's lots of work.
- With the normal
approximation you have to calculate the mean and standard
deviation, z-ify 50, and look up the area associated with
that z in the normal table and subtract that area from 1
How
large is large enough for the size of samples when deciding if the normal
approximation will be close to the real distribution? Below
are reasonable guidelines (but
they are arbitrary). Note that we consider whether or not p-hat is
close to 0.5 (central) or closer to 0 or 1.0 (the extreme values for
)
- if
~
0.5, then n can be as low as 10 or so
- if
is
close to 0 or 1.0, then if
n*
~
5 or if
sqrt[n
(1-
)] ~ 5, the n is large enough
Continuity
Correction
Note that, the binomial is discrete
(and is represented by a histogram) and the normal is continuous
and is represented by a curve.
This means that a continuity
correction is called for, especially when n is small
- First,
draw a histogram to depict the binomial probabilities and then draw a normal
curve that approximates it
- Determine which binomial probabilities
are of interest
- then
add or subtract one half to the j values you are working with
- whether you add or subtract depends
on the situation and which part of the end histogram bars are missing (assume
that the normal values split the end bars in half)
- the addition or subtraction
should be done to increase the final area under the normal
curve
Last updated September 26, 2012